We have been heads down doing the detailed and careful work to improve our CNV caller algorithm in the past three months since our we launched our Exome capable CNV caller and are very excited about the massive step forward we have made with the VarSeq 1.4.5 release.
Additionally, we have added the all new Whole Genome large-event caller capable of calling large events on whole genomes (including the cheap ultra-low read depth variety), as well as a command line tool for running all the CNV algorithms and preceding steps using a command line runner.
Along with the usual list of improvements and user requested features, this turned out to be a very significant release with lots of improvements made across the board.
The Feedback Loop of Real World Data
No algorithm developed purely on the theory of how data should look survives the first contact with the reality of the unpredictable nature of real-world data.
In the launch of our CNV algorithm for gene panel data, we worked closely with clinical partner PreventionGenetics and the validated sample data they provided was used to dial in and fire-test the algorithm.
As we expanded the scope of CNVs we called from single target to large cytogenetic events and whole chromosome aneuploidies, we had been benchmarking against CMA validated clinical samples from PreventionGenetics, as well as hundreds of myeloid leukemia tumor/normal exomes from another collaborator.
Additionally, as our value of potentially replacing expensive confirmatory methods such as MLPA and CMA with our NGS CNV caller has played out in the market. We have on boarded many more clinical labs with their own, sometimes unique gene panels. As new edge cases come up and new validated data sets can confirm new algorithmic options and improvement, we have rolled those all into this release as well.
No single detail makes or breaks an algorithm, but the ensemble of techniques applied in the latest 1.4.5 CNV target caller provides fantastic CNV calls.
In particular, we have seen vast improvements in calling large CNV events in exomes, even in tumor samples with many multiple whole chromosome deletions or duplications in a single sample.
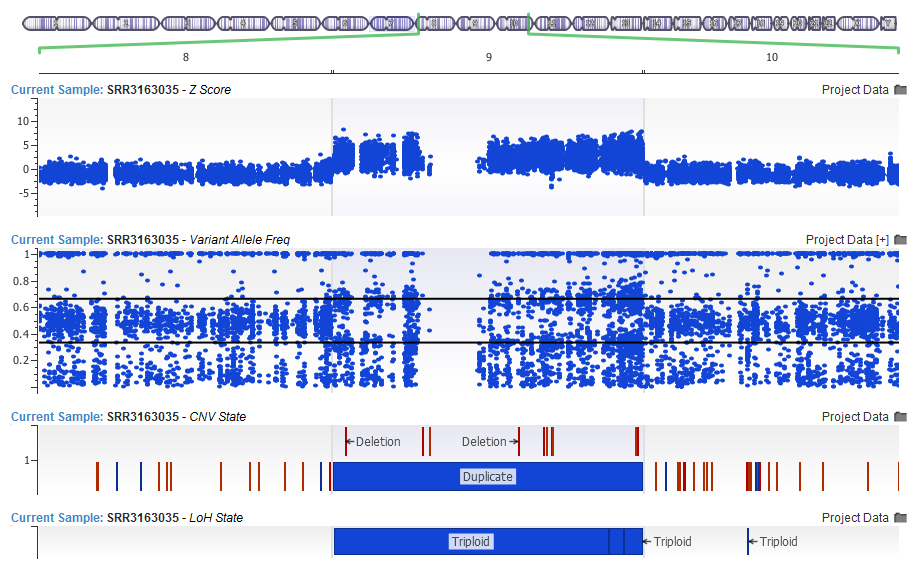
This whole chromosome duplication was also detected by the LoH caller as a Triploid region based on the Variant Allele Frequency centering around the 1/3 and 2/3 lines as apposed to the 1/2 mark expected in diploid regions.
In you are interested in calling CNVs on your gene panels or exomes, we suggest that you first run our Loss of Heterozygosity caller which looks at the Variant Allele Frequency of the imported variants.
The LoH has been updated in 1.4.5 with improved sensitivity, as well as a model for calling Triploid events. The CNV algorithm will pick this up as input, and through improved normalization make better CNV calls and report Copy Neutral LOH events.
More Flexibility to Match Your Genetic Assay
Other changes have been added to the CNV Target Caller, as well as the new CNV Binned Region (WGS) caller.
In our updated dialog, you can see we more flexibly specify a range of potential reference samples to use for normalization, as well as the option to disable the algorithms treatment of the sex (X and Y) chromosomes separately. Certain gene panels do not have enough targets in the sex chromosomes to use the algorithms strategy of normalizing them separately against gender-matched reference samples.
We have also introduced the ability to specify a set of regions to be masked for CNV calling and during target normalization. This can be helpful if there are noisy or non-clinically relevant regions that make sense to mask from the algorithm.
Additionally, we have improved the outputs of the CNV caller with the following fields:
- A p-value to give an additional quantitative value to use while assessing the quality of a CNV call. This is a student T test done between the average Z score of the event versus all targets outside events.
- A Karyotype for events that are cytoband sized. Ready for your clinical reports!
In the above screenshot, we are calling a XXY individual and other whole chromosome events on ultra-low coverage whole genomes.
One More Thing
Although the new WGS CNV caller and massive updates to the CNV infrastructure in VarSeq are the story of this release, we are always working to improve the user experience and utility of VarSeq for our many active users.
One common request we implemented was the ability to specify your own Preferred Transcript list to the gene annotation algorithm, allowing you to override the default selection of the Clinically Relevant Transcript reported in the various algorithm outputs.
Additionally, you can choose to use the abbreviated 1-letter Amino Acid encoding of the HGVS protein description instead of the default 3-letter.
There is more of course, which you can peruse in our Release Notes, but we wanted to thank our many engaged users for their feedback and suggestions as we continue the maturation of VarSeq as a clinical interpretation suite.