Now available in SVS!
Increasingly important in the analysis of the genotype to phenotype relationship is accurately accounting for the relatedness of samples. This is especially important to model correctly in plant and animal populations where man-directed breeding shapes the relationship structure.
Along with trait association, one of the high-value use cases for genotyping animals and plants is to estimate the trait-specific breeding values of samples, and the heritable effects of those SNPs.
In Golden Helix SNP and Variation Suite (SVS), the GBLUP method computes a genomic relationship matrix and from that computes the “Genomic Best Linear Unbiased Predictor” (GBLUP) of additive genetic merits by sample and of allele substitution effects (ASE) by marker.
This method has traditionally required memory proportional to the square of the number of samples being studied, putting practical limits on the samples analyzed by agrigenomic researchers.
With the release of SVS 8.5 last week, we have removed these limitations by providing a novel piece-wise approach to the matrix operations used in GBLUP and K-Fold Cross Validation.
GBLUP Without Limits
Predicting phenotypic traits from genotypes is a key focus in agrigenomics, as researchers work to increase crop yields and meat production to satisfy the needs of a growing population. Using genomic prediction tools like GBLUP enables Golden Helix SVS customers to identify the plants or animals with the best breeding potential for desirable traits without having to endure lengthy and expensive field trials.
GBLUP can be used to predict Estimated Breeding Values (EBV) for all samples in a dataset which allows for the identification of samples with the highest EBV to carry forward in breeding programs. It can also be used to identify influential loci for the phenotype of interest that can then be used for a targeted assay for diagnostic purposes.
Once the number of samples being used in GBLUP analysis exceeds 10,000, it starts to require more memory than what is reasonable in a workstation configuration. Also, the computation of eigenvalues and eigenvectors in the GBLUP calculation start to take a very long time.
Now with SVS 8.5, when running GBLUP or K-Fold Cross Validation on more than 8,000 samples, you will be presented with the following dialog:
This allows you to choose to utilize the new Large-N approximating algorithm that will only use the memory that you have available on your platform, and that may be bounded to use less compute time.
In short, we use a combination of out-of-memory scratch buffers, piece wise large data matrix operations and an adaptation of the Halko et al method for approximating matrix decompositions of large matrices using random numbers.
A trade-off in performance versus accuracy can be made, with our detailed manual providing both example run-time behaviors and expected accuracy differences of these various run-modes in Performance Tradeoffs section.
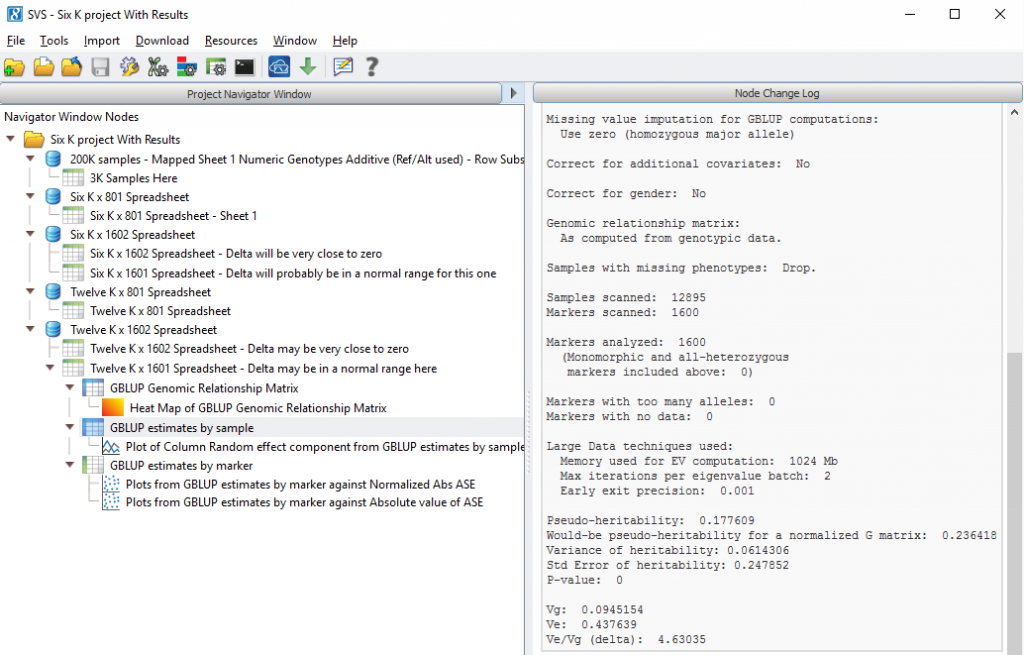
GBLUP run on large datasets now output details of the large data technique used, along with the other great run summaries provided.
At Golden Helix, we are always humbled by the continued work of our customers in their respective fields, and the relentless pushing of the bounds of the application of genomics.
With SVS 8.5, we look forward to seeing those bounds to be pushed even further.