Did you know you can analyze your Genotyping by Sequencing (GBS) data in SVS? Well you can! You can combine tools for both GWAS quality control and analysis with tools for NGS data analysis to either identify SNPs in your dataset or to identify differences between populations or sub-species.
If your species has a reference sequence or even if you have a pseudo-map for your data, you can import it into our SNP & Variation Suite software and use the numerous tools available for quality control, association tests and visualization. If you also have a gene annotation source available you can perform variant classification for your SNPs.
This blog post will walk you through an example analysis of some GBS data for Maize (Zea mays) obtained from TASSEL. If you want to follow along, you can install the SVS Viewer available here and then download the example project.
SVS supports the import of many file formats including both hapmap (*.hmp) and VCF (*.vcf). Importing imputed data is also as easy as selecting the imputed file format. In this case, the VCF file was provided, but this VCF file only had genotype data available. If your VCF file had read depth, allelic depths or quality scores, this information could be used to filter the genotypes down to high-quality genotypes. See sections 2 & 3 of the Introduction to NGS Tutorial.
When you open up the example project you should see a window that looks like Fig. 1 below.
Now there is a lot going on in this image. We’ll break it down into the following steps:
- Sample and SNP Quality Control
- Variant Classification
- Mixed Linear Model (EMMAX) Analysis
- Visualization
Sample and SNP Quality Control
To calculate sample statistics, double click on the “mdp_genotype – Genotypes(G_T) – Sheet 1” spreadsheet and go to Genotype > Genotype Sample Statistics. If you are examining the project using the SVS viewer, click on the right arrow (see Fig. 2) to expand the node and open the sample statistics results spreadsheet. 5 samples had a call rate of less than 80% and so these samples were excluded from further analysis.
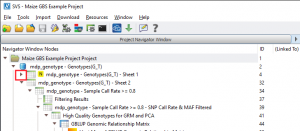
Fig. 2. Click on the indicated arrow to expand the project tree and see hidden spreadsheets (Click on images to enlarge)
SNPs were filtered by going to Genotype > Genotype Filtering by Marker and removing markers with:
- Call Rate < 0.85
- Number of alleles > 2
- Alternate Allele Frequency (MAF) < 0.05 to keep common variants
Out of 3096 SNPs, the marker filtering removed 548 that did not pass the above criteria.
Finally, in order to create the most informative kinship matrix and subset of data most useful for principal component analysis (PCA), we want to filter out markers in high LD. This is done by LD pruning, Genotype > Quality Assurance and Utilities > LD Pruning. The defaults for LD pruning were used and this further reduced the number of markers to 2130 high quality, LD pruned SNPs.
To compute the kinship or genomic relationship matrix, go to Genotype > Quality Assurance and Utilities > GBLUP Genomic Relationship Matrix. The kinship matrix can be visualized in a heat map (see Fig. 3) and it is obvious that there is cryptic relatedness between the samples or lines of Maize. In fact, we know according to the documentation from Tassel and Panzea.org that these are inbred lines.
Principal components can be computed by going to Genotype > Genotype Principal Component Analysis
Variant Classification
As this is GBS data from a VCF file, we can classify the variants based on their alternate alleles compared to the reference genome and using the location in the gene or exon. Variant classification is accessed in SVS from the genotype spreadsheet by going to DNA-Seq > Variant Classification. Use the Genotypes for Analysis spreadsheet for variant classification. These are all of the SNPs that pass quality control but have not been LD pruned. If you are interested in looking at rare variants you can use the original dataset.
This particular dataset only has 49 loss of function or missense variants in coding regions, see Fig. 4.
Mixed Linear Model (EMMAX) Analysis
This dataset has three different phenotypes, for this example, we used ear height (EarHT) as the dependent variable. Using only the measurements from location A, we ran EMMAX and linear regression by going to Genotype > Mixed Linear Model Analysis. As we already computed the kinship matrix on the filtered data, we can select that spreadsheet to use for the analysis. After setting the parameters, the dialog looked like Fig. 5. If you have additional covariates you would like to correct for or a chromosome that is Hemizygous for males, you can correct for that as well by setting the proper parameters.
Two spreadsheets of output are created, one with the results for the linear regression (fixed effects only) analysis and one with the Single-Locus Mixed Model or EMMAX results. See Fig. 6.
Visualization of Results
The output from both models can be visualized in genomic context through GenomeBrowse in SVS. There are many ways to generate a GenomeBrowse viewer in SVS, for this example, from the project navigator click on the GenomeBrowse icon. See Fig. 7.
From the GenomeBrowse window, click on the Add button in the upper left hand corner and select Project from the top left. This brings up the project tree, click on P-Values from Linear Regression (node 94) and select -log10(P-Value) from the list on the right. Do the same for P-Values from Single-Locus Mixed Model (node 97). See Fig. 8.
After renaming the titles of the plots and coloring by chromosome, the GenomeBrowse window could look like Fig. 9.
Many other tools are available for GBS data analysis in SVS, for more examples, please check out both the GWAS and DNA-Seq tutorials available for SVS. If you have any specific questions or would like to find out how SVS can aid in your data analysis please contact us at support@goldenhelix.com.