Traditionally genetic tests in cancer have focused on small gene panels that restrict their analysis to a small number of well-studied cancer genes. However, as sequencing costs have decreased, many clinical laboratories have embraced comprehensive genomic profiling tests that rely on whole exome and whole genome next-generation sequencing (NGS) workflows, which can detect millions of high-quality variants for a single sample. While the AMP Guidelines provide a robust system for the categorization of variants based on the available clinical evidence, they provide little guidance on the identification of likely driver mutations. I recently presented a webcast on this that you can also watch here.
The oncogenicity of any given variant falls along a gradient, with some variants being obvious driver mutations that are likely to enhance tumor cell proliferation, while other variants are almost certainly benign. The large number of variants detected by modern NGS workflows makes manual assessment of each individual variant’s oncogenicity impossible. This is where VarSeq’s new Cancer Classifier algorithm comes in. This algorithm can be incorporated into existing VarSeq workflows to automatically eliminate benign variants from consideration and prioritize likely oncogenic variants for interpretation following the AMP Guidelines.
During the development of our cancer scoring system, we worked with a number of different stakeholders to identify a set of criteria that can be used to quantify whether a variant is likely to be oncogenic. This classifier is based on an additive scoring system in which a variant is categorized into one of four classifications based on a set of thresholds, with scores exceeding 3 indicating an oncogenic or likely oncogenic classification, while scores below -3 indicate a benign or likely benign classification.
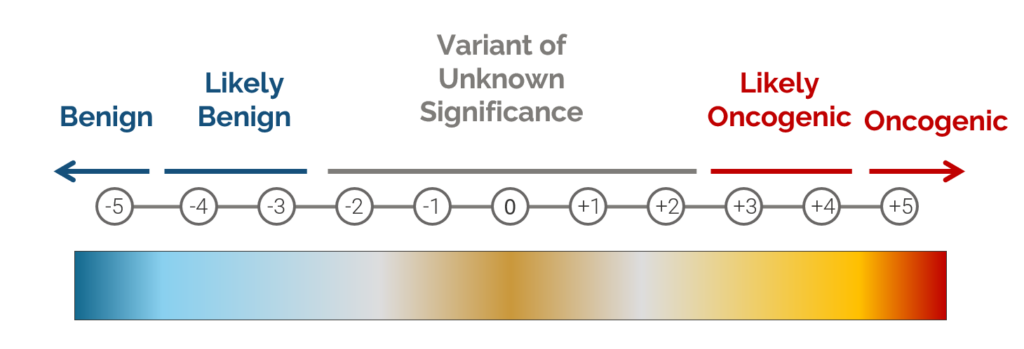
The table below shows a short summary of the criteria used to classify a variant using this scoring system. When determining if a variant is benign, the algorithm relies on things like population frequency, whether the variant is homozygous in controls, and whether the variant is an intronic variant that is predicted to have no effect on splicing. Evidence used to determine if a variant is oncogenic includes things like the variant’s occurrence in somatic catalogs and the presence of the variant in databases like CIViC or ClinVar. The algorithm also takes into account the effect of the variant. For example, when analyzing a LoF variant, the algorithm will check to see whether loss of function variants tend to be oncogenic in the gene by looking at various cancer databases.
Criteria | Score | Description |
Population Frequency (PF) | -5, -3, -1 | The variant is common in one or more population catalogs |
Homozygous in Populations (HP) | -2, -1 | The variant occurs in one or more healthy individuals with causal genotype |
In-Frame (IF) | +1 | In-frame deletions/insertions outside of repeat region |
In-silico Predictions (IP) | -1, +1 | All lines of computational evidence support a deleterious / benign effect on the gene or gene product |
Splice Predictions (SP) | +1, +2 | Multiple algorithms predict damaging splicing impact |
Somatic Catalogs (SC) | +1, +2, +3 | Rate of recurrence of mutation in somatic catalogs |
Active Region (AR) | +1 | Occurs in active binding site domain |
Hotspot Region (HR) | +1 | Occurs in cancer hotspot region |
Nearby Pathogenic (NP) | +1 | Missense variant in a region containing multiple pathogenic variants but no nearby benign variants |
Clinical Evidence (CE) | -1 +2, +3 | Previously classified as pathogenic variant or pathogenic residue |
Null Variant (NV) | +1 | LoF variant at least 50 base pairs upstream from the penultimate exon junction |
Null-Oncogenic Gene (NG) | +1 | LoF variant in a gene for which LoF variants are oncogenic |
Null Variant Downstream (ND) | +1 | LoF variant upstream from one or more previously classified pathogenic LoF variants |
Silent Variant (SV) | -3 | Synonymous mutation with no predicted impact’, ‘Synonymous mutation has predicted splicing impact |
The output of the Cancer Classifier includes a list of all criteria that were applied for each variant, along with a detailed description of why each criterion was applied. While benign variants can be easily filtered using the algorithm’s Auto Classification field, the numeric score assigned to each variant is also provided, allowing users to specify custom thresholds for excluding or prioritizing variants for interpretation.

Hopefully, this blog post has provided a useful overview of our cancer classifier and demonstrated how it can be leveraged to distinguish between benign variants and oncogenic mutations that drive tumor cell proliferation. If you have any questions or are interested in trying the algorithm out for yourself, please don’t hesitate to contact us for more information.