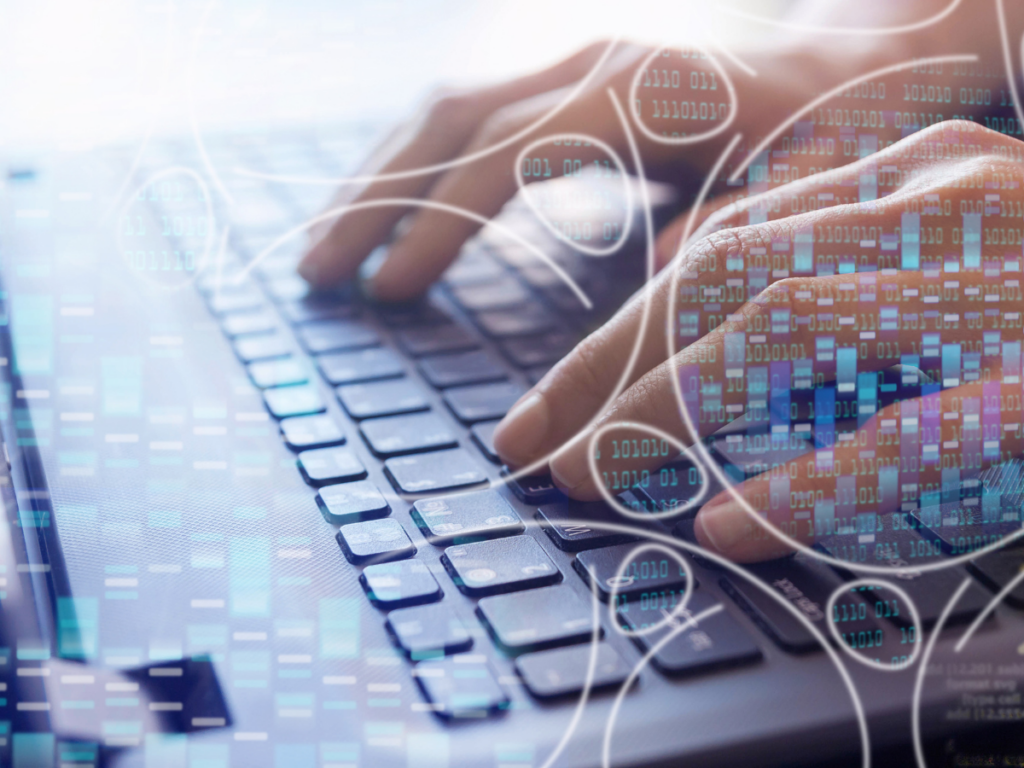
Explore the evolving landscape of genomic analysis, transitioning from targeted gene panels to whole genome sequencing.
A recent trend with our customers has been to expand their workflows from small panel sequencing analyses to larger whole exome and genome sequencing analyses. The decreasing cost of sequencing has made this a rather common request. Although more data allows for a greater variety of potential assays, it comes with the hurdle of designing and operating additional workflows. Luckily, VarSeq facilitates scaling of NGS analyses. Our recent webcast, The Wide Spectrum of Next-Generation Sequencing Assays with VarSeq, covered a number of assay types supported in VarSeq, from small cancer gene panels all the way to whole genome screens. With this blog, I wanted to summarize some of the high-level topics of workflow evolution, mainly the addition of new layers to each workflow.
Let’s start with a very simple gene panel. The amount of variants here will vary depending on the number of genes in the panel, from a few dozen variants up to several hundred, if not a few thousand, or more if there are multiple samples in the project. That may sound like a large number, but the filtering approach is going to be very straightforward (Figure 1). Below, we display a number of filter containers, organized by category, that house specific sub-filters. We generally recommend starting with variant quality filters. The quality filters here will be dependent on which fields are supplied by your secondary pipeline. Thresholds for read depth, genotype quality, and variant allele frequency may all make appearances depending on if this is a germline or somatic workflow. This has taken our variants from 26 to 22. Next, we typically recommend using population catalogs like gnomAD Exomes and 1kG Phase 3 to filter for rare variants, filtering down from 22 to just 2 rare variants. The next step would leverage RefSeq to define variants that are LOF, missense, or that interfere with splice sites. Both of the 2 variants make it through this filter. Last, we typically leverage the ACMG auto-classifier to show us which of these remaining variants are predicted to be Likely Pathogenic or Pathogenic. These variants would then be brought into VSClinical for further clinical evaluation.
The challenge, of course, comes when we step up from a handful of variants to the several hundred thousand variants we typically see in whole exome sequencing analysis. Below is our first example from a WES Breast and Ovarian Cancer Analysis (Figure 2). This is largely the same as before, with containers for variant quality, population catalogs, and gene impact. These filters, like before, do a powerful job of taking the variant count from 435,402 variants down to 3,509 at the end of the gene impact filter container. The next steps for designing the workflow are largely dependent on what is known about the sample. Leveraging either a gene panel or phenotypic matching will be most effective for reducing the variant ‘noise.’ For example, here, I used a Breast and Ovarian Gene Panel, which narrowed the scope of variants to those in the panel. This is an effective use of the tool, as I can easily go back and redesign the panel as necessary for a given sample.
The Gene Panel Manager in VarSeq allows me to effortlessly design and save alternative gene panel designs (Figure 3). For example, if I had received updated information about my patient and needed to apply a more specific gene search, I could go from a broader Breast and Ovarian Cancer panel to just the Inherited Breast Cancer and Ovarian Cancer Panel. This can be easily done with the Gene Panel Manager. Alternatively, if I needed to design a panel from scratch, I could leverage the phenotype matching option or use our integrated UK Panel App. These options make it simple to bring in patient-level information and focus a WES sample to a specific search.
As an alternative to gene panels, another popular level to WES workflows is the addition of disorder term matching (Figure 4). Here, the user can leverage the MONDO disease ontology. For example, if I were to use Huntington’s Disease, MONDO:0007739, this would narrow my search to HTT and SLC2A3.
The use of gene panels, phenotypic matching, and disease term matching is invaluable for crafting whole exome sequencing analysis workflows. Ultimately, though, we need more tools when crafting whole genome sequencing workflows. The number of variants at this stage jump from several hundred thousand to several million, highlighting the need for overall flexibility. Below, we have an example WGS workflow chain, starting at a staggering 4.7 million variants, which is effectively reduced to two potential variants for analysis (Figure 5). One of the only changes to this workflow, as opposed to the previous ones, is the addition of an inverted filtering (the NOT!) container, where we remove, for example, known benign variants from consideration. This is an extremely useful container, taking advantage of ClinVar review statuses to only remove variants that have been confirmed to be benign or likely benign by expert panels, and therefore do not need to be taken into consideration.
For this hypothetical workflow, let’s suppose we have a sample where we have a broad phenotype with a corresponding broad genes list. In this situation, we need to hone in on only the most relevant gene matches (Figure 6). For this example, using the phenotype of autism with high cognitive abilities, I would use the PhoRank Algorithm.
Here, I add a super-strict Gene Rank threshold to my workflow. This shows me only the variants in genes that are highly associated with my phenotype. After that, the ACMG classifier shows me the ten variants that are considered to be pathogenic or likely pathogenic. If you would like to know more about PhoRank, please visit our webcast on the topic.
There are several other tools we can bring in to streamline our WGS screen. These include our functional prediction tools, such as dbNSFP Functional Predictions, REVEL, CADD Scores, and more (Figure 8). These functional prediction tools are able to give us an indication of how damaging a mutation could be. We generally recommend using fairly low thresholds when utilizing the functional prediction tools because a number of known pathogenic variants have been seen to not have high functional prediction scores. For more on that topic, please see this blog.
Lastly, all of the tools described here can be brought together under a parallel filter chain approach. The advantage here is the clinician is able to look for the output of several assay types at once, filing into corresponding variant tables (Figure 9). When applied to a whole genome with several million variants, this is highly effective at directing a clinician down a route of investigation.
Overall, the leap from gene panels to whole exome sequencing and even whole genome analysis is an opportunity to build more informative workflows. This both benefits the patient with a new breadth of information to be gained and benefits the clinic, as a variety of assay types are now available for insurance reimbursement. Here we took a look at a very simple gene panel workflow and gradually added layers of filtering to direct a clinician down specific avenues of investigation. If you would like help to customize your workflows, please reach out to [email protected], and our FAS team would be happy to help you design the workflow of your dreams.