A common discussion with our customers includes the challenges with the tertiary stage of analyzing next-gen sequencing data. This is the stage where all data from gene panels, exome, or whole genome scale pass through filters to quickly isolate the clinically relevant variant contributing to a patient disorder. Golden Helix has recognized these challenges in the scale of data and filtering efficiency and worked to solve this problem with our VarSeq solution. In VarSeq, users benefit from numerous algorithms and public databases to prioritize fields leveraged in a custom filtering chain. The value here is that you can specify a filtering logic to be as conservative or sensitive as you would wish. Obviously, optimizing the filtering effectiveness is the main goal, but some panels cover many more genes than others. This makes the search more challenging when handling the sheer number of variants. A good example is a general panel for an intellectual disability which can be hundreds if not thousands of genes. Some disorders prove difficult to diagnose especially in prenatal screening or younger patients, which only opens the door to require more sensitivity when filtering through exome or whole genome data. The purpose of this blog will be to demonstrate the effectiveness of the VarSeq filtering logic for large panels by looking at an example of a likely pathogenic variant in COL4A2 found in a patient later diagnosed with porencephaly.
Starting at the beginning of this VarSeq workflow, the first filter is based on the intellectual disability panel built from the Genomics England PanelApp linked above which is a crowd-sourced tool for sharing panels which are evaluated from the scientific community. The downloaded panel overall has thousands of genes that were built into the VarSeq workflow using the Match Gene List algorithm (Figure 1). This panel “true or false” field is added to the filter chain (Figure 2) and serves as the first step. Following the panel search are filters for the quality of each variant in the sample. This includes genotype quality, removing low-quality flagged variants, and keeping variants in a heterozygous or homozygous state.
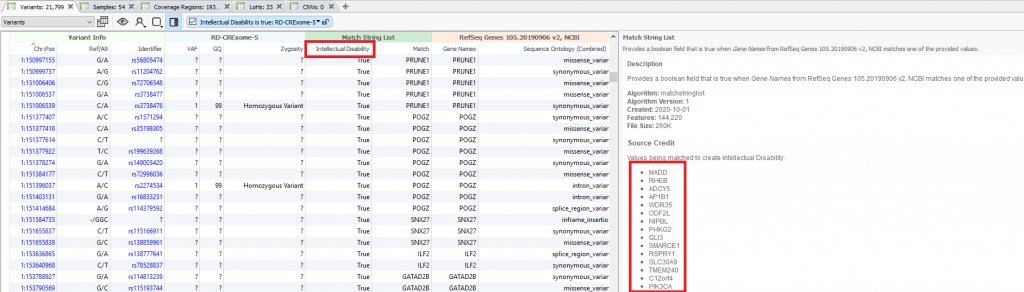

The next stage of the filter chain is to exclude common benign variants. This is done using the public annotations curated from the GHI team and supplied directly from VarSeq. In this workflow, variants present in 1kGPhase3 and gnomAD exome/genomes are removed if present in over 1% of the population. Also, a filter is made to exclude variants present in ClinVar with a high review status known with a benign classification (note the orange filter color and ! exclamation present with inverted filtering). You may have noticed the steep drop in the number of variants from the initial hundreds of thousands at the top of the filter to mere hundreds under ClinVar with only a few filtering steps!
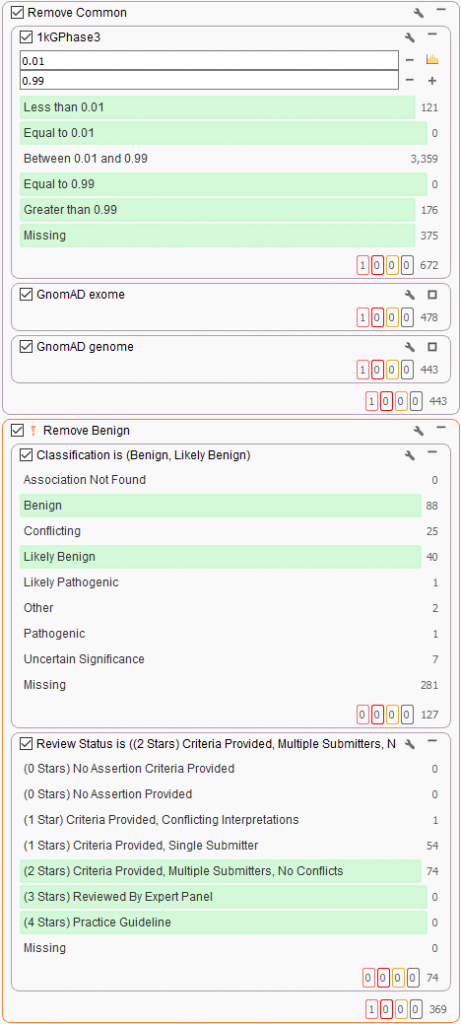
Some additional filtering then includes the capture of LoF or missense variants and splice site variants leveraging RefSeq genes. As well as the ACMG classifier integrated into VarSeq so to auto classify variants based on the Standards and Guidelines for the Interpretation of Sequence Variants.

The final result of filtering was the isolation of a likely pathogenic missense variant in exon 44 of 48 in the COL4A2 gene. From this point, the user moves into the next stages of the workflow which is the interpretation and reporting of the likely pathogenic variant.
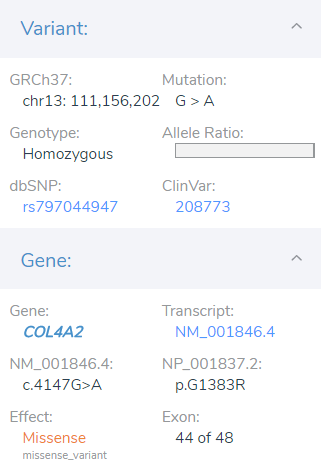
Seen in Figured 6, This G>A mutation is found to be novel amongst the population catalogs 1kGPhase 3 and gnomAD which supplies the PM2 moderate criteria. Next, the supporting PP2 criteria is also included since the COL4A2 gene is known to be sensitive to other missense variants and has a low rate of benign variants indicated with an Exome Aggregation Consortium Z score of 1.76. The second supporting criteria PP3 is included for the SIFT+PolyPhen2 predictions for damaging, and Phylop+GERP scores for being conserved. Also influential for the likely pathogenic classification is multiple submissions of this variant from Molecular Diagnostics Lab and Ambry Genetics both for Porencephaly 2. Following the NGS analysis, the initial diagnosis for the young patient was limited to intellectual disability but later confirmed for porencephaly with brain imaging.

Hopefully, this gives our current and future users insight into the power VarSeq provides in simple filtering efficiency paired with automated ACMG based interpretations processed in VSClinical. The goal here was to show how even an extremely large panel such as intellectual disability can be handled efficiently to quickly find causal variants much like this missense G>A in COL4A2. However, we do realize the value to you would be to actually test this tool for yourself. If you would like a trial version and introductory demo, please reach out to info@goldenhelix.com.